With Generative and Predictive AI, automation, data analysis, and decision-making are made possible in a variety of businesses. Two well-known paradigms in AI have surfaced: predictive AI and generative AI. They operate on separate concepts and approaches, even though they both seek to use data and algorithms for various goals. AI has become an key tool.
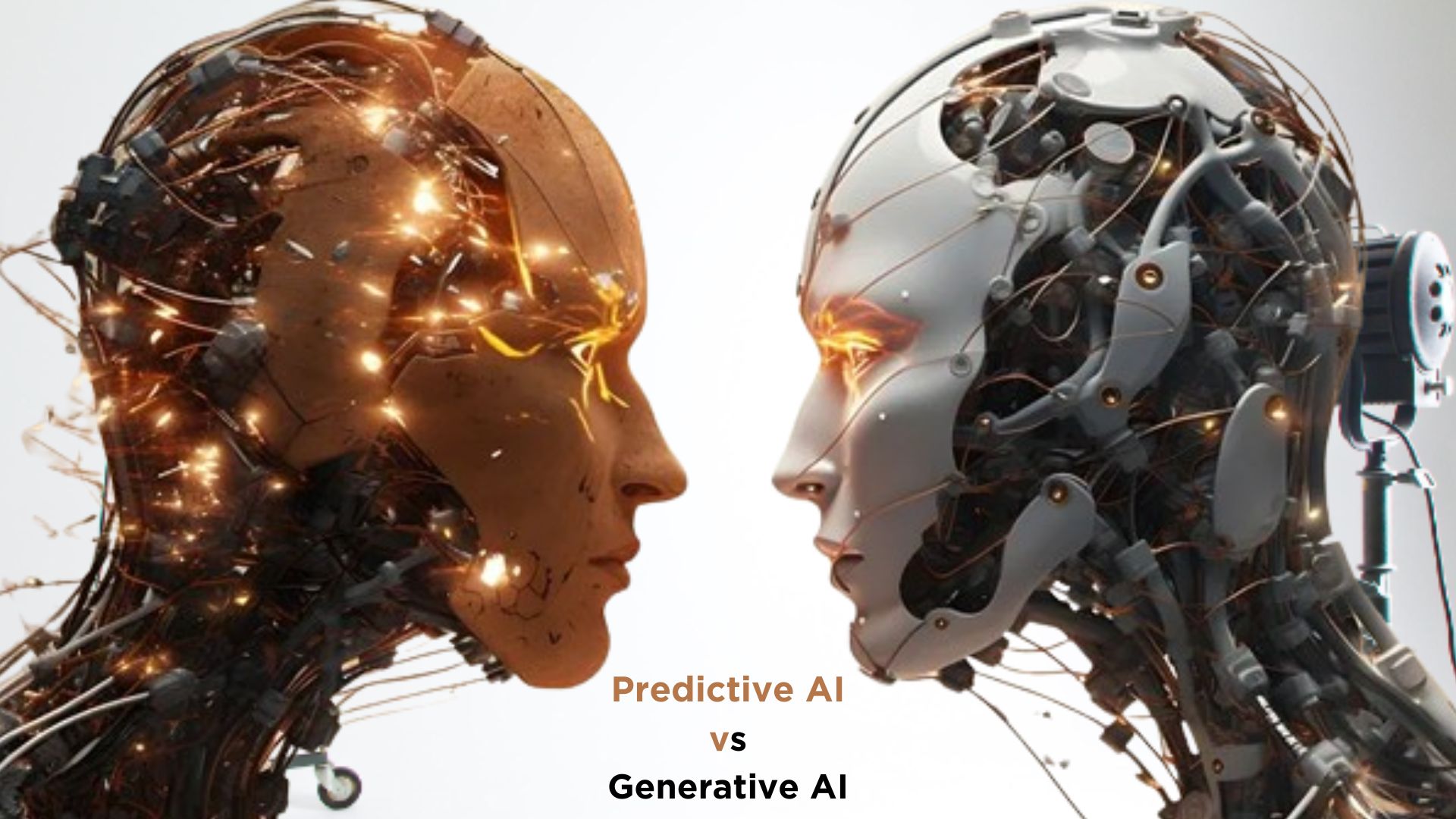
Comprehending Artificial Intelligence:
The idea of generative AI is to create fresh data or material that looks like real-world examples. This theory uses methods like deep learning, neural networks to produce innovative outputs on its own. Generational adversarial networks (GANs), in which the generator and the discriminator neural networks fight with one another to create outputs that are more and more realistic, are among the most well-known instances of generative artificial intelligence.
Benefits of AI generation:
- Originality and Creativity: Content produced by generative AI is incredibly creative and unique. Generative AI creates lifelike text and videos, realistic visuals, and musical compositions, opening up new possibilities for artistic expression.
- Data Augmentation: By producing synthetic data, generative AI can be utilized to enhance already-existing datasets.This is especially helpful in situations where obtaining assigned data is difficult or costly. Generative AI improves the generalization and resilience of machine learning models by producing synthetic examples.
- Simulation and Prediction: Complex scenario simulation and possible outcome prediction are made possible by generative artificial intelligence. In a variety of industries,that includes gaming, healthcare, and finance, generative artificial intelligence (AI) helps with risk assessment and decision-making by producing a wide range of situations and options.
Negatives of AI generation :
- Quality and Realism: In complicated domains, generative AI models may still yield outputs that are not realistic or of high quality, even with notable breakthroughs in this area. Sophisticated algorithms and a large amount of training data are needed to generate high-fidelity material, which can be time- and resource-consuming.
- Ethical Concerns: When it comes to producing fake news, misinformation, or deepfakes, the capacity of generative AI to produce realistic-looking but synthetic content presents ethical questions. Strong safeguards, rules, and moral principles are needed to address these issues and stop abuse and manipulation.
- Fairness and Bias: Generative AI models are vulnerable to biases in the training data, which might reinforce societal inequalities and biases already in place. Techniques for mitigating bias, algorithmic transparency, and careful data selection are necessary to ensure fairness and diversity in generated material.
Also Read this :https://makesyoushock.com/dell-inspiron-laptops/
Investigating Predictive AI :
Conversely, predictive AI forecasts future trends or outcomes by studying historical data. To find patterns and relationships in datasets, this paradigm makes use of data mining, machine learning algorithms, and statistical techniques. Models of predictive artificial intelligence (AI) forecast future events with precision by leveraging historical data.
Benefits of AI prediction:
- Decision Support: Predictive AI offers insightful information and aids in decision-making. Predictive AI helps in businesses to make decisions by predicting the future patterns and results.
- Risk management: Predictive AI reduces risks by anticipating possible dangers and openings. Predictive models in finance examine past data and market trends to estimate stock values, identify fraudulent activity, and efficiently manage investment portfolios.
- Personalization and Optimization: Personalized recommendations and optimizations catered to specific needs and preferences are made possible by predictive artificial intelligence. Predictive models are used in e-commerce to evaluate client data and provide tailored product recommendations that improve user experience and boost conversion rates.
Negatives of AI prediction:
- Data Availability and Quality: Historical data’s accessibility and quality are crucial components of predictive AI models. Predictive analytics systems can be rendered less successful by skewed or insufficient data, which can produce predictions that are not correct and untrustworthy insights.
- Overfitting and Generalization: Overfitting occurs when predictive AI models become so adept at memorizing training data that they fail to identify underlying patterns. Regularization, cross-validation, and feature engineering are among the approaches needed to guarantee the generalization and resilience of predictive models.
- Interpretability and Transparency: Complex predictive AI models, such as deep neural networks, frequently exhibit interpretability and transparency issues.It helps to understand the selection and prediction process of predictive models in order to build authority, clarify results, and identify potential biases.
A Comparison between Predictive and Generative AI :
Although using data and algorithms to make decisions is the general goal of both Generative AI and Predictive AI, their approaches, goals, and uses are different. Generative AI emphasizes creativity and innovation by producing fresh material or data that resembles real-world instances. On the other hand, Predictive AI prioritizes accuracy and dependability when analyzing previous data to anticipate or predict future events.
Key Differences:
- Goal: Generative AI seeks to produce new information, whereas Predictive AI is more concerned with projecting future patterns or outcomes.
- Methodology: Generative AI operates on unsupervised learning principles, whereas Predictive AI predominantly relies on supervised learning techniques.
- Applications: Predictive AI is widely used in risk management, decision assistance, and personalization, whereas generative AI is applied in simulation, creative arts, and data augmentation.
Key Similarities:
- Data and Algorithms: To do their respective jobs, both Predictive AI and Generative AI rely on data and algorithms.
- Machine Learning Techniques: Neural networks, deep learning, and statistical modeling are a few of the machine learning techniques used by both paradigms.
- Impact and Implications: Ethics, justice, and accountability are just a few of the societal areas and businesses that both predictive and generative artificial intelligence have a significant impact on.
Prospects and Difficulties :
Researchers and practitioners are faced with a multitude of opportunities and challenges as Generative AI and Predictive AI continue to progress.An attempt has made to enhance the robustness, and fairness of prediction models in the field of predictive artificial intelligence. To build confidence and trust in predictive analytics systems,There is potential to overcome current biases and limits in predictive modeling through developments that has been explained in AI.
Among the several artificial intelligence frameworks, generative AI and predictive AI are two separate approaches. Whereas Predictive AI seeks to predict future events based on past data, Generative AI concentrates on producing new material or data that resembles real-world instances.